GPT-4: Facts, Rumors and Expectations about next-gen AI model
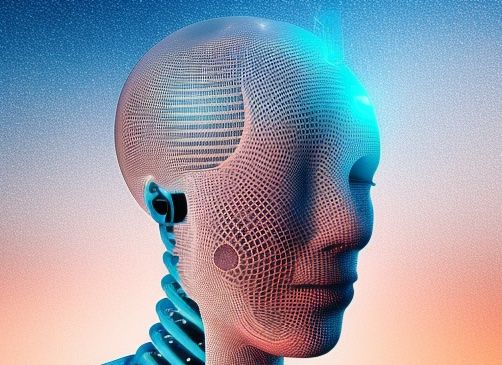
GPT-4 is the fourth version of the GPT, one of the most advanced and anticipated AI models. GPT-3 had a significant boost in performance compared to GPT-2. If GPT-4 can take the AI model to the next level, it will be like a quantum leap in capability. And the great thing is that GPT-4 is almost ready. We will likely be able to experience GPT-4 in the next few months. It should be ready in early 2023.
The concrete specifics of GPT-4 specifications are still unknown because OpenAI, the company behind GPT-4, doesn't disclose much information about the model.
In this article, I want to summarize what we know about GPT-4 so far—facts, rumors, and general expectations about the next-generation AI model.
What is GPT?
Before diving into details, it's essential to describe what a GPT is. GPT (Generative Pre-trained Transformer) is a text generation AI model trained on the data available on the internet. GPT is designed to generate human-like text.
Think of GPT as on-demand intelligence. You can use it whenever you need to solve problems that typically require human involvement.
The applications of GPT models are endless. It can be used for questions & answers, text summarization, translation, classification, code generation, etc. Some people believe that, in perspective, GPT or a similar AI model can replace Google.
GPT provides a lot of opportunities for business owners. It's possible to fine-tune the model on specific data to achieve excellent results in a particular domain (a procedure known as transfer learning). Startups and enterprise companies will use GPT as a foundation for the products they create, and it will save them from the need to train their own AI models.
What is the GPT-4 model size? What number of parameters will it have?
A parameter is a configuration variable that is internal to the AI model and whose value can be extracted from the provided data. AI models use parameters when making predictions.
A number of parameters that an AI model has is a commonly used metric of performance. The Scaling Hypothesis states that language modeling performance improves smoothly and predictably as we appropriately scale up a model size, data, and computational power. That's why many AI model creators were focused on increasing the number of parameters their models have.
Since 2018 when GPT-1 was released, OpenAI has followed the "the bigger, the better" strategy. GPT-1 had 117 million parameters, GPT-2 had 1.2 billion parameters, and GPT-3 raised the number even further to 175 billion parameters. It means that the GPT-3 model has 100 times more parameters than GPT-2. GPT-3 is a very large model size, with 175 billion parameters.
In an August 2021 interview with Wired, Andrew Feldman, founder and CEO of Cerebras, a company that partners with OpenAI to train the GPT model, mentioned that GPT-4 will be about 100 trillion parameters. It might seem like GPT-4 will be 100x more powerful than GPT-3.
100 trillion parameters is a low estimate for the number of neural connections in the human brain. If GPT-4 will have 100 trillion parameters, it will match the human brain in terms of parameters.
No surprise that this made people so excited.
In reality, model size is not directly related to the quality of the outcome it produces. The number of parameters does not necessarily correlate with the performance that an AI model has. It's only one factor that impacts the model performance. Right now, we have much larger AI models than GPT-3, but they aren't the best in terms of performance. For example, Megatron-Turing NLG, built by Nvidia and Microsoft, has more than 500B parameters, and it's the largest model right now. But despite that MT-NLG isn't the best in terms of performance. The smaller model can reach higher performance levels.
Also, the larger the model, the more expensive to fine-tune it. GPT3 was hard enough and expensive to train, but if you increase the model's size by 100x, it will be extremely expensive in terms of computational power and the amount of training data required for the model.
There Is a low chance that OpenAI will have 100T parameters in GPT-4 because just increasing the number of training parameters won't cause any drastic improvement if training data is also not increased proportionally. Large models are often unoptimized (take Megatron-Turing NLG as an example). It is very expensive to train the model, and often companies have to make a trade between AI model accuracy and the cost of training. For example, GPT-3 was trained only once, and, despite the errors in the AI model, OpenAI wasn't able to train the model again due to unaffordable costs.
It all means that OpenAI will likely start to avoid the "bigger is better" approach and focus on the quality of the model itself. Most likely, GPT-4 would be roughly GPT -3's size.
What is more interesting is that OpenAI will likely shift the focus toward other aspects that impact the model performance, such as algorithms and alignment. GPT-4 might be the first large AI model with sparsity at its core. Sparse models use conditional computation to reduce computing costs—not all neurons in the AI model are active at any given time. The model can easily scale beyond a trillion parameters without incurring high computing costs. Sparse models also better understand the context—they can keep a lot more choices of "next word/sentence" based on what the user provided. As a result, sparse models are more similar to actual human thinking than their predecessors.
Will GPT-4 be a text-only model or multimodal?
AI models can be text-only or multimodal. Text-only models accept the text as input and produce text as output. GPT-3 is a text-only model. The multimodal model accepts text, audio, image, and even video inputs. It gives users the ability to use AI to generate audiovisual content. Multimodality is the future of AI because the world we live in is multimodal. DALL-E is a multimodal model.
Good multimodal models are significantly harder to build than good language-only models because multimodal models should be able to combine textual and visual info into a single representation. As far as I can see, OpenAI is trying to find the limits that language-only models have, and they will likely continue moving in this direction with GPT-4 rather than trying to create a powerful multimodal model. So GPT-4 will likely be a text-only model.
Will GPT-4 be less dependent on good prompting?
Anyone who has experience working with GPT-3 knows how important good prompting is. When you struggle to find the right prompt, the end result won’t be good enough. One expectation that many people have about GPT-4 is that this model will be less dependent on good prompting, giving users more freedom to formulate the intention in the way they want it and be more confident that the system will understand them.
The goal that OpenAI pursues is to make language models follow human intentions. High chance that the GPT-4 will be more aligned than GPT-3. Prior to releasing the ChatGPT, the company invested in InstructGPT, a GPT-3 model trained on human feedback to follow instructions. InstructGPT is trained on a large dataset of instructional texts, including recipe instructions, how-to guides, and other types of written instructions. The goal of InstructGPT is to generate natural language text that is clear, concise, and easy to follow.
Will GPT-4 revolutionize the world?
Will GPT-4 impact society in the same way as Covid?
GPT4 will be out soon and will probably cause a similar economic shock to one from Covid. Instant distribution with nearly instant adoption and nearly instant productivity increase for hundreds of millions of knowledge workers. Brace yourselves, 2023 is coming
— Nick Davidov (@Nick_Davidov) December 24, 2022
GPT-4 will definitely impact how people do their work, but it doesn't mean that that impact will be compared with Covid (at least during the first few years). Most likely, GPT-4 will give a boost to productivity so that people will be able to complete work with AI faster than without it. At the same time, we are only at the beginning of the adoption of AI tools, AI tools should earn the trust of people, and only after that people will start using them in daily work.
Personal experience. I've been using ChatGPT, an advanced chatbot based on the GPT-3.5 language model, for work since the day it was publicly available, and I should say that it provides many benefits. Yet, the output that this tool generates often requires validation and correction. For example, the tool can make up things or use incorrect references. Sometimes it takes more time to create text using ChatGPT than to write it myself from scratch. The model hasn't yet achieved a human-like understanding of the nuances and complexities of real-life experience.
Even if GPT-4 offers a quantum leap in terms of performance, the system's adoption rate won't be noticeable during the first year. It will take a few years for the general audience to adopt the change. And at the beginning, we will likely have many areas where humans will be remarkably better.
References: Algorithmic Bridge (Alberto Romero), Reddit (OpenAI thread), Wired (Andrew Feldman interview), AI Breakfast, Greylock (Sam Altman Interview).